nginx+flume网络流量日志实时数据分析实战
网络流量日志数据分析-概述
除了政府和公益类网站之外,大多数网站的目的都是为了产生货币收入,说白了就是赚钱。要创建出用户需要的网站就必须进行网站分析,通过分析,找出用户实际需求,构建出符合用户需求的网站。 网站分析,可以帮助网站管理员、运营人员、推广人员等实时获取网站流量信息,并从流量来源、网站内容、网站访客特性等多方面提供网站分析的数据依据。从而帮助提高网站流量,提升网站用户体验,让访客更多的沉淀下来变成会员或客户,通过更少的投入获取最大化的收入。
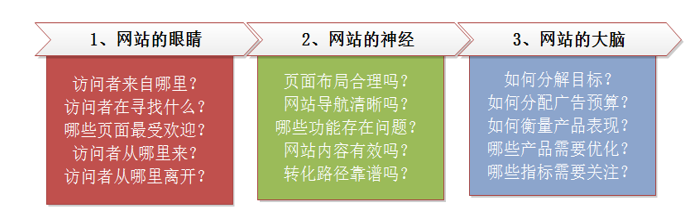
网络流量日志数据分析-数据处理流程
网站流量日志数据分析整体流程基本上就是依据数据的处理流转流程进行。通俗可以概括为:数据从哪里来和数据到哪里去,可以分为以下几个大的步骤:
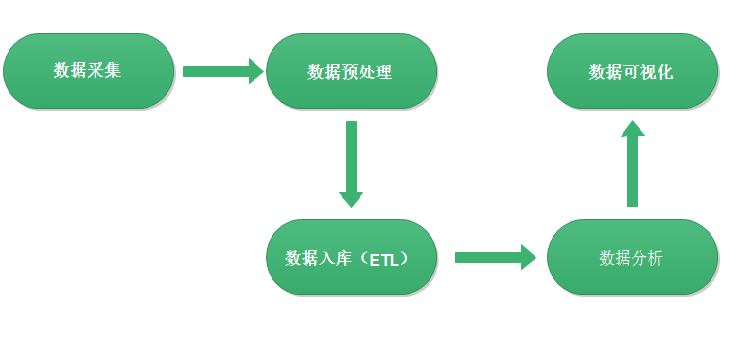
网络流量日志数据分析-数据采集
网站日志文件
记录网站日志文件的方式是最原始的数据获取方式,主要在服务端完成,在网站的应用服务器配置相应的写日志的功能就能够实现,很多web应用服务器自带日志的记录功能。如Nginx的access.log日志等。
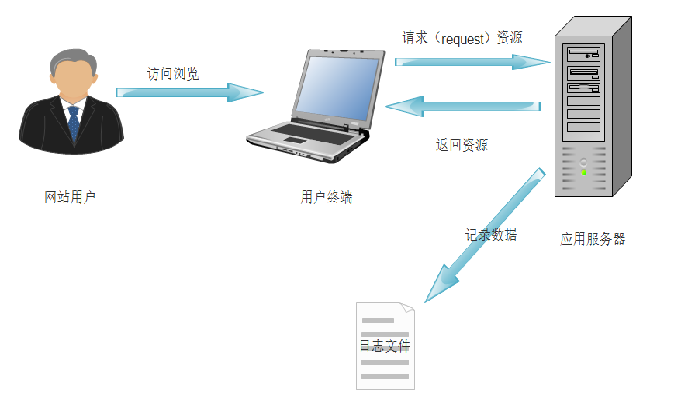
启动nginx服务器:
/usr/local/nginx/sbin/nginx
/usr/local/nginx/sbin/nginx -s stop #停止服务器。
通过浏览器访问: http://192.168.88.100/
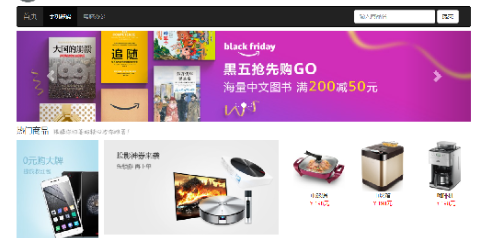
刷新页面,查看日志信息:
[root@node1 logs]# tail -f /usr/local/nginx/logsaccess.log
192.168.88.1 - - [03/Feb/2021:16:50:15 +0800] "GET /img/zy03.jpg HTTP/1.1" 200 90034 "http://192.168.88.100/" "Mozilla/5.0 (Windows NT 10.0; Win64; x64) AppleWebKit/537.36 (KHTML, like Gecko) Chrome/87.0.4280.66 Safari/537.36"
192.168.88.1 - - [03/Feb/2021:16:50:15 +0800] "GET /img/title2.jpg HTTP/1.1" 200 1703 "http://192.168.88.100/" "Mozilla/5.0 (Windows NT 10.0; Win64; x64) AppleWebKit/537.36 (KHTML, like Gecko) Chrome/87.0.4280.66 Safari/537.36"
Chrome/87.0.4280.66 Safari/537.36"
日志字段解释
1、访客ip地址: 58.215.204.118
2、访客用户信息: - -
3、请求时间:[18/Sep/2018:06:51:35 +0000]
4、请求方式:GET
5、请求的url:/wp-includes/js/jquery/jquery.js?ver=1.10.2
6、请求所用协议:HTTP/1.1
7、响应码:304
8、返回的数据流量:0
9、访客的来源url:http://blog.fens.me/nodejs-socketio-chat/
10、访客所用浏览器:Mozilla/5.0 (Windows NT 5.1; rv:23.0) Gecko/20100101 Firefox/23.0
网络流量日志数据分析-数据采集-Flume框架
Flume概述
Flume是Cloudera提供的一个高可用的,高可靠的,分布式的海量日志采集、聚合和传输的软件。 Flume的核心是把数据从数据源(source)收集过来,再将收集到的数据送到指定的目的地(sink)。为了保证输送的过程一定成功,在送到目的地(sink)之前,会先缓存数据(channel),待数据真正到达目的地(sink)后,flume在删除自己缓存的数据。 当前Flume有两个版本。Flume 0.9X版本的统称Flume OG(original generation),Flume1.X版本的统称Flume NG(next generation)。由于Flume NG经过核心组件、核心配置以及代码架构重构,与Flume OG有很大不同,使用时请注意区分。改动的另一原因是将Flume纳入 apache 旗下,Cloudera Flume 改名为 Apache Flume。
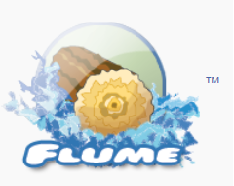
Flume运行机制
Flume系统中核心的角色是agent,agent本身是一个Java进程,一般运行在日志收集节点。
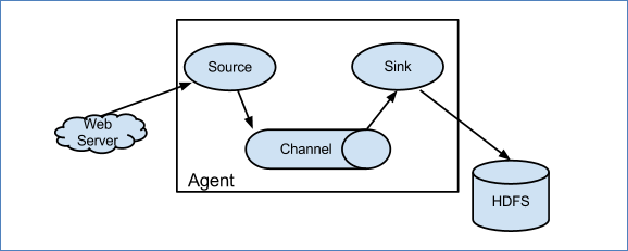
每一个agent相当于一个数据传递员,内部有三个组件: Source:采集源,用于跟数据源对接,以获取数据; Sink:下沉地,采集数据的传送目的,用于往下一级agent传递数据或者往 最终存储系统传递数据; Channel:agent内部的数据传输通道,用于从source将数据传递到sink;
Flume安装部署
课程提供的虚拟机中已经安装好了flume。
Flume采集
现将日志数据采集到HDFS上.
# Name the components on this agent a1.sources = r1 a1.sinks = k1 a1.channels = c1
Describe/configure the source
#a1.sources.r1.type = exec
#a1.sources.r1.command = tail -F /usr/local/nginx/logs/access.log
#a1.sources.r1.channels = c1
a1.sources = r1
a1.sources.r1.type = TAILDIR
a1.sources.r1.channels = c1
a1.sources.r1.positionFile = /var/log/flume/taildir_position.json
a1.sources.r1.filegroups = f1
a1.sources.r1.filegroups.f1 = /usr/local/nginx/logs/access*.log
…..
运行flume采集
/export/server/flume-1.8.0/bin/flume-ng agent -c conf -f /export/server/flume-1.8.0/conf/web_log.conf -n a1 -Dflume.root.logger=INFO,console
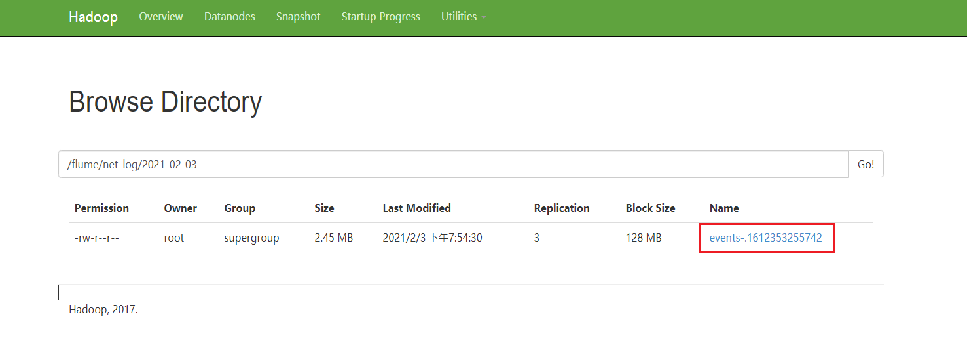
数据预处理-清洗
Flume采集获取的日志数据是不能直接用于分析的,需要做下预处理:
预处理需求:
1:剔除字段长度不够的日志数据
2:剔除对分析没有意义的字段
3:转换日期格式
4:对原日志数据进行切割,分隔符指定为 ‘\001’
5:对数据的有效行进行标记
数据清洗:
hadoop jar /export/data/mapreduce/web_log.jar cn.itcast.bigdata.weblog.pre.WeblogPreProcess
网络流量日志数据分析-点击流模型数据
点击流概念
点击流(Click Stream)是指用户在网站上持续访问的轨迹。注重用户浏览网站的整个流程。用户对网站的每次访问包含了一系列的点击动作行为,这些点击行为数据就构成了点击流数据(Click Stream Data),它代表了用户浏览网站的整个流程。
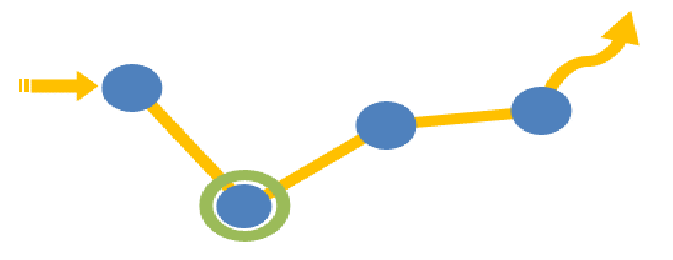
在点击流模型中,存在着两种模型数据:PageViews、Visits。
点击流模型pageviews
Pageviews模型数据专注于用户每次会话(session)
的识别,以及每次session内访问了几步和每一步的停留时间。
在网站分析中,通常把前后两条访问记录时间差在30分钟以内算成一次会话
。如果超过30分钟,则把下次访问算成新的会话开始。
大致步骤如下:
在所有访问日志中找出该用户的所有访问记录
把该用户所有访问记录按照时间正序排序
计算前后两条记录时间差是否为30分钟
如果小于30分钟,则是同一会话session的延续
如果大于30分钟,则是下一会话session的开始
用前后两条记录时间差算出上一步停留时间
最后一步和只有一步的 业务默认指定页面停留时间60s。
--得到pageviews模型
hadoop jar /export/data/mapreduce/web_log.jar cn.itcast.bigdata.weblog.clickstream.ClickStreamPageView
点击流模型Visits
Visits模型专注于每次会话session内起始、结束的访问情况信息。比如用户在某一个会话session内,进入会话的起始页面和起始时间,会话结束是从哪个页面离开的,离开时间,本次session总共访问了几个页面等信息。
大致步骤如下:
在pageviews模型上进行梳理
在每一次回收session内所有访问记录按照时间正序排序
第一天的时间页面就是起始时间页面
业务指定最后一条记录的时间页面作为离开时间和离开页面。
得到visits模型
hadoop jar /export/data/mapreduce/web_log.jar cn.itcast.bigdata.weblog.clickstream.ClickStreamVisit
网络日志数据分析-数据加载
对于日志数据的分析,Hive也分为三层:ods层、dw层、app层
创建数据库
create database if not exists web_log_ods;
create database if not exists web_log_dw;
create database if not exists web_log_app;
创建ODS层数据表
原始日志数据表
drop table if exists web_log_ods.ods_weblog_origin;
create table web_log_ods.ods_weblog_origin(
valid string , --有效标记
remote_addr string, --访客ip
remote_user string, --访客用户信息
time_local string, --请求时间
request string, --请求url
status string, --响应状态码
body_bytes_sent string, --响应字节数
http_referer string, --来源url
http_user_agent string --访客终端信息
)
partitioned by (dt string)
row format delimited fields terminated by '\001';
点击流模型pageviews表
drop table if exists web_log_ods.ods_click_pageviews;
create table web_log_ods.ods_click_pageviews(
session string, --会话id
remote_addr string, --访客ip
remote_user string, --访客用户信息
time_local string, --请求时间
request string, --请求url
visit_step string, --访问步长
page_staylong string, --页面停留时间(秒)
http_referer string, --来源url
http_user_agent string,--访客终端信息
body_bytes_sent string,--响应字节数
status string --响应状态码
)
partitioned by (dt string)
row format delimited fields terminated by '\001';
点击流visits模型表
drop table if exists web_log_ods.ods_click_stream_visits;
create table web_log_ods.ods_click_stream_visits(
session string, --会话id
remote_addr string, --访客ip
inTime string, --会话访问起始时间
outTime string, --会话访问离开时间
inPage string, --会话访问起始页面
outPage string, --会话访问离开页面
referal string, --来源url
pageVisits int --会话页面访问数量
)
partitioned by (dt string)
row format delimited fields terminated by '\001';
表数据加载
load data inpath '/output/web_log/pre_web_log' overwrite into table web_log_ods.ods_weblog_origin partition(dt='2021-02-01');
load data inpath '/output/web_log/pageviews'overwrite into table web_log_ods.ods_click_pageviews partition(dt='2021-02-01');
load data inpath '/output/web_log/visits' overwrite into table web_log_ods.ods_click_stream_visits partition(dt='2021-02-01');
网络日志数据分析-明细表、宽表实现
概念
事实表的数据中,有些属性共同组成了一个字段(糅合在一起),比如年月日时分秒构成了时间,当需要根据某一属性进行分组统计的时候,需要截取拼接之类的操作,效率极低。
为了分析方便,可以事实表中的一个字段切割提取多个属性出来构成新的字段,因为字段变多了,所以称为宽表,原来的成为窄表。
又因为宽表的信息更加清晰明细,所以也可以称之为明细表。
drop table web_log_dw.dw_weblog_detail;
create table web_log_dw.dw_weblog_detail(
valid string, --有效标识
remote_addr string, --来源IP
remote_user string, --用户标识
time_local string, --访问完整时间
daystr string, --访问日期
timestr string, --访问时间
month string, --访问月
day string, --访问日
hour string, --访问时
request string, --请求的url
status string, --响应码
body_bytes_sent string, --传输字节数
http_referer string, --来源url
ref_host string, --来源的host
ref_path string, --来源的路径
ref_query string, --来源参数query
ref_query_id string, --来源参数query的值
http_user_agent string --客户终端标识
)
partitioned by(dt string)
row format delimited fields terminated by '\001';
通过查询插入数据到明细宽表 dw_weblog_detail中,这里需要借助Hive中的内置函数parse_url_tuple对url进行解析,将以下sql存入: /export/data/hive_sql/web_log_detail.sql中
insert into table web_log_dw.dw_weblog_detail partition(dt='2021-02-01')
select c.valid,c.remote_addr,c.remote_user,c.time_local,
substring(c.time_local,1,10) as daystr,
substring(c.time_local,12) as tmstr,
substring(c.time_local,6,2) as month,
substring(c.time_local,9,2) as day,
substring(c.time_local,12,2) as hour,
c.request,c.status,c.body_bytes_sent,c.http_referer,c.ref_host,c.ref_path,c.ref_query,c.ref_query_id,c.http_user_agent
from
(SELECT
a.valid,a.remote_addr,a.remote_user,a.time_local,
a.request,a.status,a.body_bytes_sent,a.http_referer,a.http_user_agent,b.ref_host,b.ref_path,b.ref_query,b.ref_query_id
FROM web_log_ods.ods_weblog_origin a LATERAL VIEW
parse_url_tuple(regexp_replace(http_referer, """, ""), 'HOST', 'PATH','QUERY', 'QUERY:id') b as ref_host, ref_path, ref_query,
ref_query_id) c;
执行sql抽取转换字段到中间表明细表 t_ods_tmp_detail hive -f ‘/export/data/hive_sql/web_log_detail.sql’
网络日志数据分析-APP层数据指标开发
基础指标分析
--浏览页面次数(pv)
select count(*) as pvs from web_log_dw.dw_weblog_detail where valid = true and dt='2021-02-01';--独立访客(uv)
select count(distinct remote_addr) as uvs from web_log_dw.dw_weblog_detail where valid = true and dt='2021-02-01';
--访问次数(vv)
select count(session) from ods_click_stream_visits where dt='2021-02-01'
基础指标入库
--基础指标入库
drop table if exists web_log_app.app_webflow_basic_info;
create table web_log_app.app_webflow_basic_info(date_val string,pvs bigint,uvs bigint,vvs bigint) partitioned by(dt string);--允许笛卡尔积
set spark.sql.crossJoin.enabled=true;
insert into table web_log_app.app_webflow_basic_info partition(dt='2021-02-01')
select '2021-02-01',a.,b. from
(
select count(*) as pvs,count(distinct remote_addr) as uvs from web_log_dw.dw_weblog_detail where dt='2021-02-01'
) a
join
(
select count(session) as vvs from web_log_ods.ods_click_stream_visits where dt='2021-02-01'
) b;
基础指标分析-多维度分析
--计算该处理批次(一天)中的各小时pvs
drop table web_log_app.app_pvs_everyhour_oneday;
create table web_log_app.app_pvs_everyhour_oneday(month string,day string,hour string,pvs bigint) partitioned by(dt string);insert into table web_log_app.app_pvs_everyhour_oneday partition(dt='2021-02-01')
select a.month as month,a.day as day,a.hour as hour,count(*) as pvs from web_log_dw.dw_weblog_detail a
where a.dt='2021-02-01' group by a.month,a.day,a.hour;--计算每天的pvs
drop table web_log_app.app_pvs_everyday;
create table web_log_app.app_pvs_everyday(pvs bigint,month string,day string);
insert into table web_log_app.app_pvs_everyday
select count(*) as pvs,a.month as month,a.day as day from web_log_dw.dw_weblog_detail a
group by a.month,a.day;
复合指标分析
--复合指标统计分析
--人均浏览页数(平均访问深度)
--需求描述:统计今日所有来访者平均请求的页面数。
--总页面请求数pv/去重总人数uvdrop table web_log_app.app_avgpv_user_everyday;
create table web_log_app.app_avgpv_user_everyday(
day string,
avgpv string);--方式一:
insert into table web_log_app.app_avgpv_user_everyday
select '2021-02-01',pv/uv from web_log_app.app_webflow_basic_info;--方式二:
insert into table web_log_app.app_avgpv_user_everyday
select '2021-02-01',sum(b.pvs)/count(b.remote_addr) from
(select remote_addr,count(*) as pvs from web_log_dw.dw_weblog_detail where dt='2021-02-01' group by remote_addr) b;--平均访问时长
--平均每次访问(会话)在网站上的停留时间。 --体现网站对访客的吸引程度。 --平均访问时长=访问总时长/访问次数。
--先计算每次会话的停留时长
select session, sum(page_staylong) as web_staylong from web_log_ods.ods_click_pageviews where dt='2021-02-01'
group by session;
--计算平均访问时长
select
sum(a.web_staylong)/count(a.session)
from
(select session, sum(page_staylong) as web_staylong from web_log_ods.ods_click_pageviews where dt='2021-02-01'
group by session) a;
复合指标分析-topn
--热门页面统计
--统计最热门的页面top10drop table web_log_app.app_hotpages_everyday;
create table web_log_app.app_hotpages_everyday(day string,url string,pvs string);--方式1
insert into table web_log_app.app_hotpages_everyday
select '2021-02-01',a.request,a.request_counts from
(select request as request,count(request) as request_counts
from web_log_dw.dw_weblog_detail where dt='2021-02-01' group by request having request is not null
) a
order by a.request_counts desc limit 10;
--方式2
insert into table web_log_app.app_hotpages_everyday
select * from
(
SELECT
'2021-02-01',a.request,a.request_counts,
RANK() OVER( ORDER BY a.request_counts desc) AS rn
FROM
(
select request as request,count(request) as request_counts
from web_log_dw.dw_weblog_detail where dt='2021-02-01' group by request having request is not null
)a
)b
where b.rn <= 10
;
复合指标分析-漏斗模型-转化分析
转化,指网站业务流程中的一个封闭渠道,引导用户按照流程最终实现业务目标(比如商品成交);在这个渠道中,我们希望访问者一路向前,不要回头也不要离开,直到完成转化目标。漏斗模型则是指进入渠道的用户在各环节递进过程中逐渐流失的形象描述。
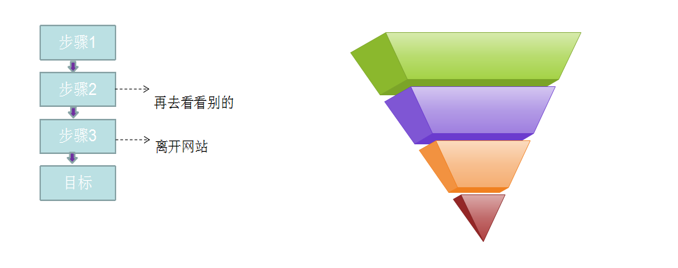
需求分析
在一条指定的业务流程中,求出各个步骤的完成人数及相对上一个步骤的百分比。
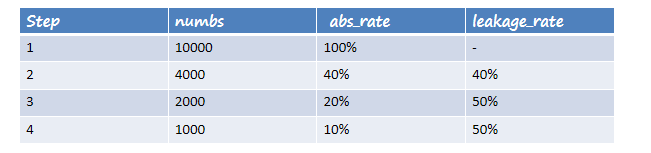
定义好业务流程中的页面标识,下例中的步骤为:
Step1、 /item
Step2、 /category
Step3、 /index
Step4、 /order
load data local inpath '/export/data/hivedatas/click-part-r-00000' overwrite into table web_log_ods.ods_click_pageviews2 partition(dt='2021-02-01');
---1、查询每一个步骤的总访问人数
UNION All将多个SELECT语句的结果集合并为一个独立的结果集
create table web_log_app.app_oute_numbs as
select 'step1' as step,count(distinct remote_addr) as numbs from web_log_ods.ods_click_pageviews where dt='2021-02-01' and request like '/item%'
union all
select 'step2' as step,count(distinct remote_addr) as numbs from web_log_ods.ods_click_pageviews where dt='2021-02-01' and request like '/category%'
union all
select 'step3' as step,count(distinct remote_addr) as numbs from web_log_ods.ods_click_pageviews where dt='2021-02-01' and request like '/order%'
union all
select 'step4' as step,count(distinct remote_addr) as numbs from web_log_ods.ods_click_pageviews where dt='2021-02-01' and request like '/index%';
查询结果:
+---------------------+----------------------+--+
| dw_oute_numbs.step | dw_oute_numbs.numbs |
+---------------------+----------------------+--+
| step1 | 1029 |
| step2 | 1029 |
| step3 | 1028 |
| step4 | 1018 |
+---------------------+----------------------+--+
–2、查询每一步骤相对于路径起点人数的比例
--级联查询,自己跟自己join
select rn.step as rnstep,rn.numbs as rnnumbs,rr.step as rrstep,rr.numbs as rrnumbs from web_log_app.app_oute_numbs rn
inner join
web_log_app.app_oute_numbs rr;
自join后结果如下图所示:
+---------+----------+---------+----------+--+
| rnstep | rnnumbs | rrstep | rrnumbs |
+---------+----------+---------+----------+--+
| step1 | 1029 | step1 | 1029 |
| step2 | 1029 | step1 | 1029 |
| step3 | 1028 | step1 | 1029 |
| step4 | 1018 | step1 | 1029 |
| step1 | 1029 | step2 | 1029 |
| step2 | 1029 | step2 | 1029 |
| step3 | 1028 | step2 | 1029 |
| step4 | 1018 | step2 | 1029 |
| step1 | 1029 | step3 | 1028 |
| step2 | 1029 | step3 | 1028 |
| step3 | 1028 | step3 | 1028 |
| step4 | 1018 | step3 | 1028 |
| step1 | 1029 | step4 | 1018 |
| step2 | 1029 | step4 | 1018 |
| step3 | 1028 | step4 | 1018 |
| step4 | 1018 | step4 | 1018 |
+---------+----------+---------+----------+--+
--每一步的人数/第一步的人数==每一步相对起点人数比例
select tmp.rnstep,tmp.rnnumbs/tmp.rrnumbs as abs_rate
from
(
select
from
web_log_app.app_oute_numbs t1
join
web_log_app.app_oute_numbs t2
on t2.step = 'step1';
) tmp
tmp
+---------+----------+---------+----------+--+
| rnstep | rnnumbs | rrstep | rrnumbs |
+---------+----------+---------+----------+--+
| step1 | 1029 | step1 | 1029 |
| step2 | 1029 | step1 | 1029 |
| step3 | 1028 | step1 | 1029 |
| step4 | 1018 | step1 | 1029 |
–3、查询每一步骤相对于上一步骤的漏出率
--首先通过自join表过滤出每一步跟上一步的记录
select rn.step as rnstep,rn.numbs as rnnumbs,rr.step as rrstep,rr.numbs as rrnumbs from web_log_app.app_oute_numbs rn
inner join
web_log_app.app_oute_numbs rr
where cast(substr(rn.step,5,1) as int)=cast(substr(rr.step,5,1) as int)-1;注意:cast为Hive内置函数 类型转换
select cast(1 as float); --1.0
select cast('2016-05-22' as date); --2016-05-22
+---------+----------+---------+----------+--+
| rnstep | rnnumbs | rrstep | rrnumbs |
+---------+----------+---------+----------+--+
| step1 | 1029 | step2 | 1029 |
| step2 | 1029 | step3 | 1028 |
| step3 | 1028 | step4 | 1018 |
+---------+----------+---------+----------+--+
–然后就可以非常简单的计算出每一步相对上一步的漏出率
select tmp.rrstep as step,tmp.rrnumbs/tmp.rnnumbs as abs_rate
from
(
select rn.step as rnstep,rn.numbs as rnnumbs,rr.step as rrstep,rr.numbs as rrnumbs from web_log_app.app_oute_numbs rn
inner join
web_log_app.app_oute_numbs rr) tmp
where cast(substr(tmp.rnstep,5,1) as int)=cast(substr(tmp.rrstep,5,1) as int)-1;
创建结果表
drop table if exists web_log_app.app_bounce_rate;
create table web_log_app.app_bounce_rate
(
step_num string,
numbs bigint,
abs_rate double,
leakage_rate double
);
insert into table web_log_app.app_bounce_rate
select abs.step,abs.numbs,abs.rate as abs_rate,rel.leakage_rate as leakage_rate
from
(
select tmp.rnstep as step,tmp.rnnumbs as numbs,tmp.rnnumbs/tmp.rrnumbs * 100 as rate
from
(
select rn.step as rnstep,rn.numbs as rnnumbs,rr.step as rrstep,rr.numbs as rrnumbs from web_log_app.app_oute_numbs rn
inner join
web_log_app.app_oute_numbs rr) tmp
where tmp.rrstep='step1'
) abs
left outer join
(
select tmp.rrstep as step,tmp.rrnumbs/tmp.rnnumbs * 100 as leakage_rate
from
(
select rn.step as rnstep,rn.numbs as rnnumbs,rr.step as rrstep,rr.numbs as rrnumbs from web_log_app.app_oute_numbs rn
inner join
web_log_app.app_oute_numbs rr) tmp
where cast(substr(tmp.rnstep,5,1) as int)=cast(substr(tmp.rrstep,5,1) as int)-1
) rel
on abs.step=rel.step;
创建mysql结果表
CREATE DATABASE web_log_result;
drop table if exists web_log_result.app_webflow_basic_info;
CREATE TABLE web_log_result.app_webflow_basic_info(MONTH VARCHAR(50),DAY VARCHAR(50),pv BIGINT,uv BIGINT,ip BIGINT,vv BIGINT);
--Sqoop数据导出
/export/server/sqoop-1.4.7/bin/sqoop export
--connect jdbc:mysql://192.168.88.100/web_log_result
--username root
--password 123456
--table app_webflow_basic_info
--input-fields-terminated-by '\001'
--export-dir /user/hive/warehouse/web_log_app.db/app_webflow_basic_info/dt=2021-02-01