理论上的参数量
之前翻译了 Christopher Olah 的那篇著名的 Understanding LSTM Networks,这篇文章对于整体理解 LSTM 很有帮助,但是在理解 LSTM 的参数数量这种细节方面,略有不足。本文就来补充一下,讲讲如何计算 LSTM 的参数数量。
建议阅读本文前先阅读 Understanding LSTM Networks 的原文或我的译文。
首先来回顾下 LSTM。一层 LSTM 如下:

这里的xt 实际上是一个句子的 embedding(不考虑 batch 维度),shape 一般为 [seq_length, embedding_size]
。图中的A 就是 cell,xt 中的词依次进入这个 cell 中进行处理。可以看到其实只有这么一个 cell,所以每次词进去处理的时候,权重是共享的,将这个过程平铺展开,就是下面这张图了:

实际上我觉得这里 x t x_t xt 并不准确,第一个 x t x_t xt 应该指的是整句话,而第二个 x t x_t xt 应该指的是这句话中最后一个词,所以为了避免歧义,我认为可以将第一个 x t x_t xt 重命名为 x x x,第二个仍然保留,即现在 x x x 表示一句话,该句话有 t + 1 t+1 t+1 个词, x t x_t xt 表示该句话的第 t + 1 t+1 t+1 个词, t ∈ [ 0 , t ] t \in [0, t] t∈[0,t]。
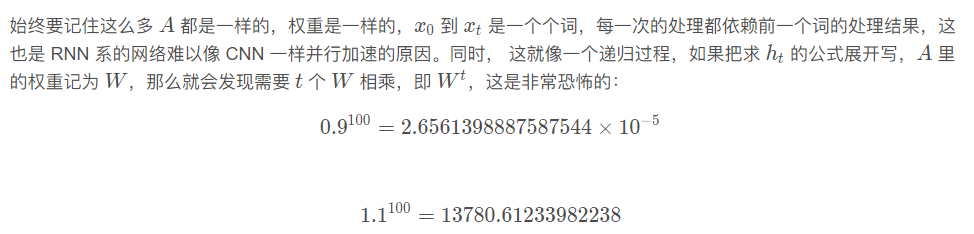
一个不那么小的数被多次相乘之后会变得很小,一个不那么大的数被多次相乘之后会变得很大。所以,这也是普通 RNN 容易出现梯度消失/爆炸的问题的原因。
扯远了点。

(embedding_size + hidden_size) * hidden_size + hidden_size
一个 cell 有 4 个这样结构相同的网络,那么一个 cell 的总参数量就是直接 × 4:
((embedding_size + hidden_size) * hidden_size + hidden_size) * 4
注意这 4 个权重可不是共享的,都是独立的网络。
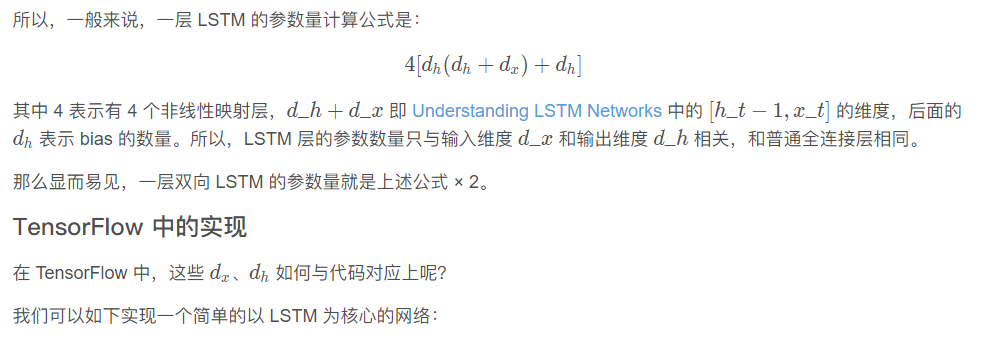
import tensorflow as tf
model = tf.keras.model.Sequential(
tf.keras.layers.Embedding(1000, 128),
tf.keras.layers.LSTM(units=64),
tf.keras.layers.Dense(10)
)
model.summary()
输入如下:
Model: "sequential"
Layer (type) Output Shape Param #
embedding_1 (Embedding) (None, None, 128) 128000
lstm_1 (LSTM) (None, 64) 49408
dense_1 (Dense) (None, 10) 650
Total params: 178,058
Trainable params: 178,058
Non-trainable params: 0
_________________________________________________________________
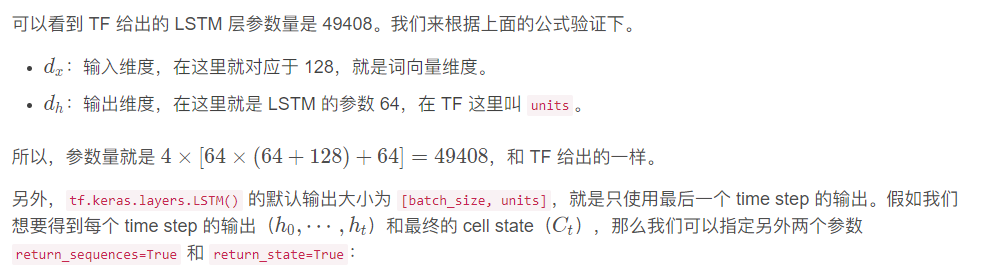
inputs = tf.random.normal([64, 100, 128]) # [batch_size, seq_length, embedding_size]
whole_seq_output, final_memory_state, final_carry_state = tf.keras.layers.LSTM(64, return_sequences=True, return_state=True)(inputs)
print(f"{whole_seq_output.shape=}")
print(f"{final_memory_state.shape=}")
print(f"{final_carry_state.shape=}")
输出:
whole_seq_output.shape=TensorShape([32, 100, 64]) # 100 表示有 100 个词,即 100 个 time step
final_memory_state.shape=TensorShape([32, 64])
final_carry_state.shape=TensorShape([32, 64])
OK,LSTM 的参数量应该挺清晰了,欢迎在评论区留下你的想法。
Reference
- Counting No. of Parameters in Deep Learning Models by Hand
- deep learning - Number of parameters in an LSTM model - Data Science Stack Exchange
- machine learning - How to calculate the number of parameters of an LSTM network? - Stack Overflow
- tensorflow - In Keras, what exactly am I configuring when I create a stateful
LSTM
layer with Nunits
? - Stack Overflow - 理解 LSTM 网络 · Alan Lee
- Recurrent Neural Networks (RNN) with Keras | TensorFlow Core
- LSTM is dead. Long Live Transformers! - YouTube