类器官智能(OI):生物计算和容器中智能的新前沿
Recent advances in human stem cell-derived brain organoids promise to replicate critical molecular and cellular aspects of learning and memory and possibly aspects of cognition in vitro. Coining the term “organoid intelligence” (OI) to encompass these developments, we present a collaborative program to implement the vision of a multidisciplinary field of OI. This aims to establish OI as a form of genuine biological computing that harnesses brain organoids using scientific and bioengineering advances in an ethically responsible manner. Standardized, 3D, myelinated brain organoids can now be produced with high cell density and enriched levels of glial cells and gene expression critical for learning. Integrated microfluidic perfusion systems can support scalable and durable culturing, and spatiotemporal chemical signaling. Novel 3D microelectrode arrays permit high-resolution spatiotemporal electrophysiological signaling and recording to explore the capacity of brain organoids to recapitulate the molecular mechanisms of learning and memory formation and, ultimately, their computational potential. Technologies that could enable novel biocomputing models via stimulus-response training and organoid-computer interfaces are in development. We envisage complex, networked interfaces whereby brain organoids are connected with real-world sensors and output devices, and ultimately with each other and with sensory organ organoids (e.g. retinal organoids), and are trained using biofeedback, big-data warehousing, and machine learning methods. In parallel, we emphasize an embedded ethics approach to analyze the ethical aspects raised by OI research in an iterative, collaborative manner involving all relevant stakeholders. The many possible applications of this research urge the strategic development of OI as a scientific discipline. We anticipate OI-based biocomputing systems to allow faster decision-making, continuous learning during tasks, and greater energy and data efficiency. Furthermore, the development of “intelligence-in-

云原生|K8S 网络篇
Before diving into the details on how packets flow inside a Kubernetes cluster, let's first clear up the requirements for a Kubernetes network.
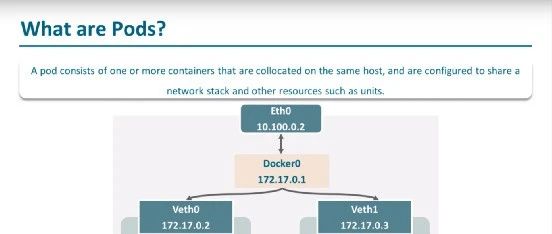
绘制韦恩图及计算P值
这里的a为A数据集的基因数,b为B数据集的基因数,inter为两者交集的基因数。
计算韦恩图P值的代码为
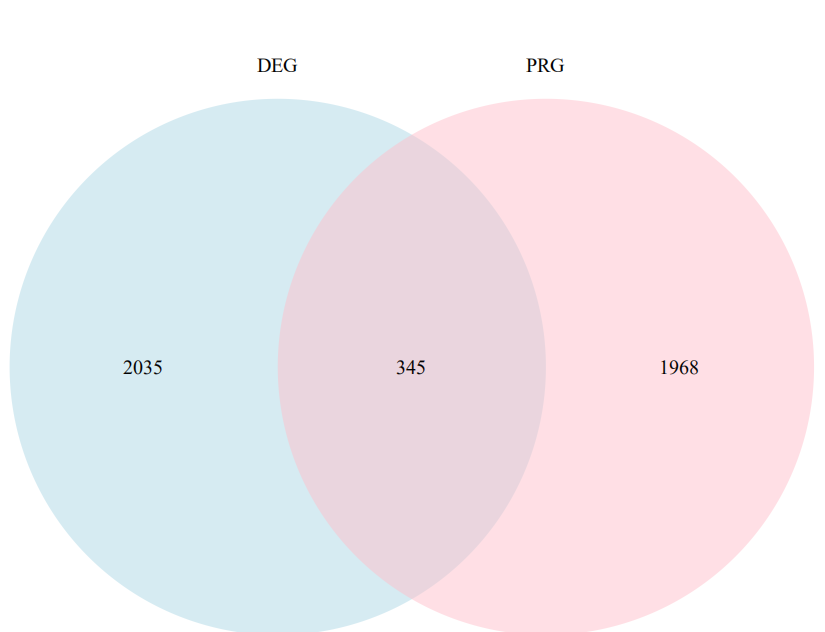
【Python】我的Python学习笔记【1】【using Python 2】
简单总结functools.partial的作用就是,把一个函数的某些参数给固定住(也就是设置默认值),返回一个新的函数,调用这个新函数会更简单。
C# 截取图片斜距形
C# 截取图片斜距形需要:从一张大图中截取出某一区域的图片前提:.Net framework 4.8 及以上示例代码:代码语言:javascript复制private static void ImageCutRectangle()
{
// 打开待截取的大图
using (Image seatImg = Image.FromFile("4.jpg"))
{
// 这里是截取出的图片的宽度高...
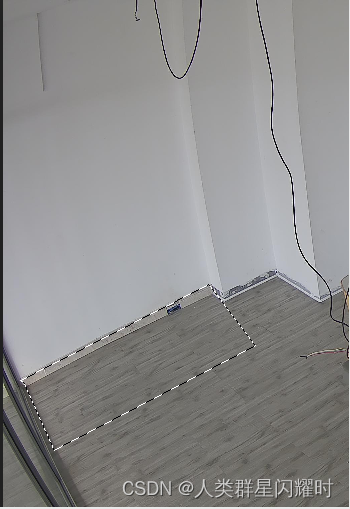
阅读笔记|Verifying and Monitoring IoTs Network Behavior Using MUD Profiles
info: A. Hamza, D. Ranathunga, H. H. Gharakheili, T. A. Benson, M. Roughan, and V. Sivaraman, “Verifying and Monitoring IoTs Network Behavior Using MUD Profiles,” IEEE Trans. Dependable and Secure Comput., vol. 19, no. 1, pp. 1–18, Jan. 2022, doi: 10.1109/TDSC.2020.2997898.